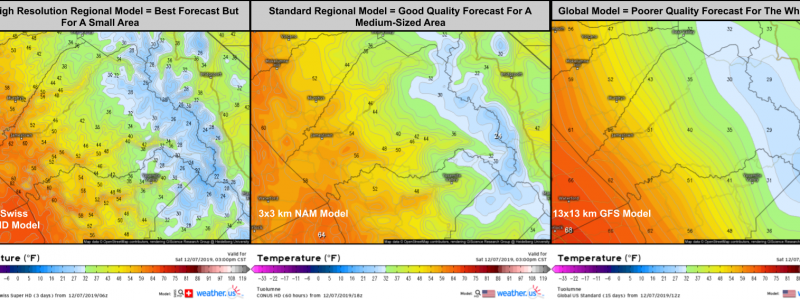
Model Mania: What’s The Difference Between Global and Regional Weather Models?
Hello everyone!
This post is the second in the our Model Mania series which hopes to shed some light on weather models without getting too far into the advanced mathematics that makes this subject inaccessible to many. The first post in the series gave a basic overview of weather models, the process of running them, and the sources of error in their predictions. While you don’t need to read that post to understand this one, I recommend taking a look if you’re interested. This post will discuss the difference between global and regional models including the strengths and weaknesses of both.
Why bother running two different types of weather model? These models require an incredible amount of computational power to solve the governing equations of the atmosphere at various points in the atmosphere. Not only would a hypothetically ‘perfect’ (we already know from part one that this isn’t possible due to observational and theoretical constraints) model need to run these calculations at every single point on the earth’s surface, it would also need to run the calculations for every single point in the atmosphere above the surface. Given that the computer code used to run these calculations rings up somewhere in the millions of lines, you might be able to generate a perfect 5 forecast but the computer wouldn’t finish it for two weeks. That’s not very helpful!
To get around this problem, we only solve the governing equations at certain points in the atmosphere, ignoring the space between the points. This enables the forecast to be completed before the weather we’re trying to predict actually happens. However, there is an important tradeoff in this process. If you pick fewer points for your model to calculate, your forecast will be done faster, but it will be less accurate. Choose more points and you’ll have a better forecast but it will take much longer.
Currently, the points most global weather models calculate the governing equations for are separated by roughly 10km or 6.2 miles horizontally, with a wide variety of vertical distances between layers of points. This grid spacing (distance between points) is great for figuring out where features like hurricanes, nor’easters, and warm/cold fronts are headed. However, other features such as severe thunderstorms can fit entirely between four points such that the model has no idea they might exist. If we want to predict where individual thunderstorm cells might be going, we need a lot more points.
Unfortunately, if we were to run a model for the entire world with enough points to see every thunderstorm cell, it wouldn’t finish making its forecast until it was much too late. The solution to this problem is to run a regional model at a high resolution (so we can see the thunderstorms) but only for a small geographic region. After all, we can feel pretty confident that predicting the development of thunderstorms six hours from now in Oklahoma doesn’t require knowledge of thunderstorms over Brazil, China, Africa, or the intervening swaths of ocean. Keep in mind that if we wanted to know what thunderstorms might develop over Oklahoma next week, we would need to know about the weather in far-flung corners of the world, but because we’re only interested in a very short term forecast (regional models only forecast for the next 1-3 days), we can afford to neglect a large part of the earth’s atmosphere in favor of more detailed analysis of a smaller area.
Now that we know what the purpose of a regional model is and what tradeoffs are involved are required to achieve its higher resolution, we can discuss how to use both global and regional models most effectively.
Global models, while lacking in specificity, are best equipped to forecast large-scale features such as mid latitude storm systems and major heat waves/cold snaps. They’re also the best (and as of this writing, the only) way to forecast weather patterns more than 3-5 days into the future. Regional models are best used for localized predictions such as tomorrow’s high temperature in your town (vs the next town over which may be influenced by terrain or bodies of water invisible to the global models) or the approximate location of individual storm cells. Despite their much higher resolution, the limitations I discussed in the first article of this series (lack of observations and certain equations) mean that even the regional models can’t predict exactly which towns will be hit by severe storms, though they’re now generally accurate to within a county or two.
Despite their tradeoffs and limitations, both regional and global models are valuable tools for weather prediction. Like all weather models, they are merely tools with a specific useful purpose and can lead you astray if used improperly. You won’t get very far trying to forecast individual thunderstorm cells using global model output, just like regional models can’t tell you whether there might be a significant cold snap five days from now.
Hopefully by now you have a better understanding of the differences between regional and global models and what each is most useful for.
The next article in the Model Mania series will discuss the two most famous global models, the ECMWF and the GFS and why despite their overlapping missions, both have an important role in weather forecasting.
-Jack