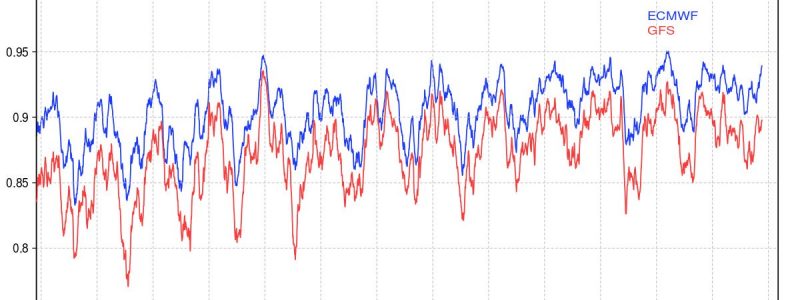
Model Mania: What Are The ECMWF and GFS Models, and Why Are They Different?
Hello everyone!
This post is the third in our Model Mania series which hopes to give a brief introduction to weather models for those without a rigorous atmospheric science background. In the previous two posts, I explained what exactly weather models are/how they work and the difference between regional and global weather models. This post will take a deeper dive into the two most famous weather models, the GFS and ECMWF. These are both global models, which means they’re both trying to serve the same purpose (predict large scale weather patterns 3-10 days in advance), but how and why are they different?
The GFS is the global model run by the US Government under the leadership of the National Oceanic and Atmospheric Administration (NOAA) and its subsidiary agencies. The GFS model is funded by American taxpayers, which means its forecast output is freely available to anyone who wants it. If you want the raw output data fresh from the GFS model, you can download it for free off NOAA’s website. While the raw data is available for free, making that data useful to end users requires a substantial amount of post-processing where the raw data is ‘massaged’ into a format more recognizable to humans than the strings of 1’s and 0’s produced by the model itself. The maps and graphs you see at weather.us and weathermodels.com are produced using post-processing algorithms our meteorologists and programmers have developed.
The ECMWF is also a global model, but instead of being run by the US Government, it is run by an independent intergovernmental entity supported by 34 European nations. Note that the nomenclature here is a bit confusing, but ECMWF stands for the European Center for Medium-Range Weather Forecasts and is the name of both the organization and the model. The way ECMWF the organization is set up means that they can and do charge for their forecast data, though international law dictates that some of it (classified as WMO-Essential) is available for the public good. If you go to most free weather model sites, the only ECMWF data you’ll see is WMO-Essential, which only includes a small handful of parameters at a very low resolution. As of this writing, we are not aware of any site besides ours that lets you view full-resolution ECMWF data for free. If you want the raw forecast output from the ECMWF, you’ll have to pay for it and then apply your post-processing algorithms to make it usable for humans. Alternatively, you could let us take care of that for you and view the latest ECMWF output at weather.us or weathermodels.com.
Now that you know who’s in charge of each model and how access to their information differs, you’re probably wondering how their forecasts are different. The above graphic shows a comparison of the ECMWF (left) and GFS (right) six-day forecasts for the US on the evening of December 14th, 2019. I don’t yet know which model forecast will be more accurate (I’ll discuss more about the accuracy of the GFS and ECMWF models in a minute), but immediately a few key differences are visible.
First, you’ll notice the GFS model precipitation (shaded) field (field in this context means gridded dataset, or a dataset for which there is a value at many different points on a grid) is much more pixelated than the ECMWF precipitation field. This is because the GFS is run at a lower resolution than the ECMWF model, meaning it has grid points located farther apart (one is placed every 13km in the GFS model compared to every 9km in the ECMWF model). As you’ll recall from the previous installment of this series, a lower resolution generally means a less accurate forecast, as there are more atmospheric and topographic features the model is unaware of.
You’ll also notice substantial differences in the placement and structure of the storm system forecast over the Eastern US at the time in question. These differences are due to a combination of resolution differences (mentioned above), differences in data assimilation (the process of telling the model what’s happening in the atmosphere right now), and differences in the sets of governing equations each model uses to turn its given initial conditions into a forecast. Did the terms in that last sentence sound unfamiliar? Check out the first post of this series to learn about initial conditions, governing equations, and more of the basics of running a weather model.
A detailed explanation of the different governing equations (sometimes referred to as physics packages) employed by each model would require a substantial amount of advanced math, so I’ll leave the interested reader with a couple links to detailed documentation of how the latest (as of 12/9/19) ECMWF and GFS models calculate their forecasts at each gridpoint. A detailed effort to explain each model’s data assimilation process would require similar mathematics, so if you’re looking to put your knowledge of linear algebra to practical use, I’ll suggest reading up on the technique the GFS model uses for data assimilation (Ensemble Kalman Filtering). For those who are interested in a deeper dive into data assimilation but don’t want to deal with linear algebra or partial differential equations, the ECMWF has developed an excellent short course (~1 hour) that explains how their (much more advanced) data assimilation process works without getting too deep into the math.
So which model is generally speaking more accurate?
Statistically speaking, the very clear answer is that the ECMWF consistently performs better than the GFS, as the model skill score graph above shows. At no point since 2007 (and likely for a while before then) has the GFS produced an generally more accurate 5-day forecast for the Northern Hemisphere between 20 and 80N than the ECMWF. That being said, there have been many cases where the GFS has been more accurate than the ECMWF for specific storms. For example, the GFS predicted the formation of Tropical Storm Dorian long before the ECMWF did. Perhaps a more famous example was the snowstorm of January 27th, 2015 where the ECMWF forecasted over two feet of snow for New York City which would have brought the city to an absolute standstill. The GFS on the other hand predicted less than a foot, which would be disruptive but certainly not crippling. Central Park ended up recording 9.8″ of snow.
Despite the occasional ‘loss’ to the GFS, the ECMWF remains the consistent leader in medium-range weather prediction, but why?
The first part of this story is that ECMWF the organization has a much narrower range of responsibilities than NOAA does. According to their respective websites, here is a quick comparison of the mission each organization has.
Notice that 2/3 of ECMWF’s objectives relate to either producing accurate weather forecasts or conducting research for the purpose of producing more accurate weather forecasts. While weather forecasts are a crucially important part of NOAA’s mission, it is only one relatively small part of their overall responsibilities which includes a much broader range of environmental concerns.
It is extremely important to acknowledge that both these institutions are tremendously important, and provide extraordinary societal value for the relatively small costs they impart on taxpayers. My goal in explaining the organizational differences between NOAA and ECMWF is not to argue that one is better than the other, or that NOAA should be more like ECMWF or vice versa. That being said, if your goal is accurate weather forecasts, it helps to devote most of your entire organization to that specific aim. That’s not to say that Europe isn’t working on predicting changes in climate, oceans, and coasts, or working to conserve and manage its coastal and marine ecosystems, but those tasks are not left to ECMWF, which enables it to focus more intently on improving the accuracy of their weather forecasts.
Perhaps at this point you’re thinking that the organizational comparison between NOAA and ECMWF is unfair to the US precisely because NOAA is charged with such a broad range of responsibilities. However, even within the sub-agency (the Environmental Modeling Center, EMC) of the sub-agency (the National Centers for Environmental Prediction, NCEP) of NOAA devoted entirely to weather prediction, a similar pattern emerges. While the ECMWF runs one global model and 51 ensemble members (more on those to come in our ensemble series), EMC maintains a comprehensive suite of models ranging from the GFS global model to the HRRR (High Resolution Rapid Refresh) regional model to the HWRF (Hurricane Weather Research and Forecasting) hurricane-specific regional model. As a result, the pool of resources (time, money, computational power, and personnel) the US has dedicated to numerical weather prediction is split among a wide range of models, each with a specific purpose.
While this might seem inefficient, consider the value provided by these other models. The HRRR has demonstrated considerable skill in predicting when and where small-scale features like heavy snow bands and thunderstorm clusters will form, and how intense they’ll be. Additionally, the HRRR is run every hour which means that forecasters can re-evaluate their predictions based on new information much more frequently than if we had to wait for a run of the global models every six hours. When it comes to hurricanes, the ECMWF and GFS models do a fairly good job predicting where a hurricane or tropical storm might go, but are notoriously bad at figuring out how strong it will be. The HWRF model helps to fill in that gap by providing more accurate intensity forecasts that are critically important to making decisions about which parts of the coastline might need to be evacuated ahead of a storm.
While the suite of regional models supported by the US Government’s weather prediction system has limited the accuracy of our global model, the GFS, it enables a much more comprehensive view of our atmosphere that ECMWF isn’t designed to provide. Of course, the real win-win-win for weather prediction here in the US would be to have enough time, money, and computational power set aside to weather modelling in the US so we can have both a global model that matches ECMWF’s skill and a full suite of specialized/regional models, but you’ll have to take that up with your congressional representatives.
While this post was on the longer and text-heavier side, I hope it has been helpful to your understanding of what the GFS and ECMWF models are, how they’re different, and why the ECMWF is generally more skillful than the GFS. In the next Model Mania post, I’ll explain why both models are needed despite the fact that the GFS consistently lags behind the ECMWF in terms of performance.
-Jack
Very informative article! Thank you for sharing!
This is a very informative article with a great deal of useful information, explained in an easy-to-understand way.
Note: Nore recently,in 2021, GFS was more accurate than ECMWF.
Fascinating post. Enjoyed it a lot and look forward to reading past and future posts. Thank you for helping me better understand weather and weather models.